As the first year of EU Taxonomy reporting concludes and organisations gear up for the Corporate Sustainability Reporting Directive (CSRD), a crucial lesson has emerged—efficiently collecting and standardising ESG data is paramount. Companies often encounter challenges in consolidating ESG data from various IT systems (ERPs, Financials, HR, carbon accounting, etc.), resulting in inconsistencies across entities and organisational layers. To tackle these data quality issues, it has become crucial to adopt a system that can gather and standardise ESG data into a unified data model. This article explores the benefits of implementing an ESG Data Library powered by a Data Lake architecture to address these challenges.
Data Management Platforms: Data Warehouse vs Data Lake vs Data Lakehouse
With the rise of Cloud hosting services and with an increasing amount of data needing to be stored, companies tend to be overrun with data and faced with data quality issues. The need emerged for them to centralise, synthesise, and cleanse the data before they can transform it into actionable insights. Data management platforms were developed to fulfil this need.
Before we explore how the concept of a data lake applies to ESG data, we will first explore what is meant by the different terms “Data Warehouse”, “Data Lake”, and “Data Lakehouse”.
Data Warehouse
Data warehouses are used by organisations as a unified repository to store data sets from various sources, but these are only able to support structured data. Structured data uses the structured query (SQL) programming language, is stored in SQL relational databases, and it is easily accessible via applications such as Enterprise Resource Planning (ERPs). As the data is already structured, data warehouses are easily accessible for ad-hoc analysis by business users.
Data Lake
A data lake allows storing and handling large amounts of structured, but also semi-structured (i.e.: CSV, JSON, XML) and unstructured data in natural, raw format (i.e. Word, PDFs, images). The data is copied “as is” from the source to the data lake and then transformed subsequently based on what needs to be analysed. Data lakes typically benefit data scientists and engineers who can work with data in its raw form to gain new insights.
Data Lakehouse
The most recent type of data management architecture is called data lakehouse: it combines the robust structure and data management features of a data warehouse with the low-cost storage, scalability, and flexibility features of a data lake. Data lakehouses are excellent platforms for advanced analytics, machine learning, and data science tools.
While the data lakehouse offers the most advanced features, based on companies’ reporting requirements and the complexity of the data sources, either of these solutions can be applied to an ESG data management system. For the purpose of the article, the terms data lake and data lakehouse will be used interchangeably.
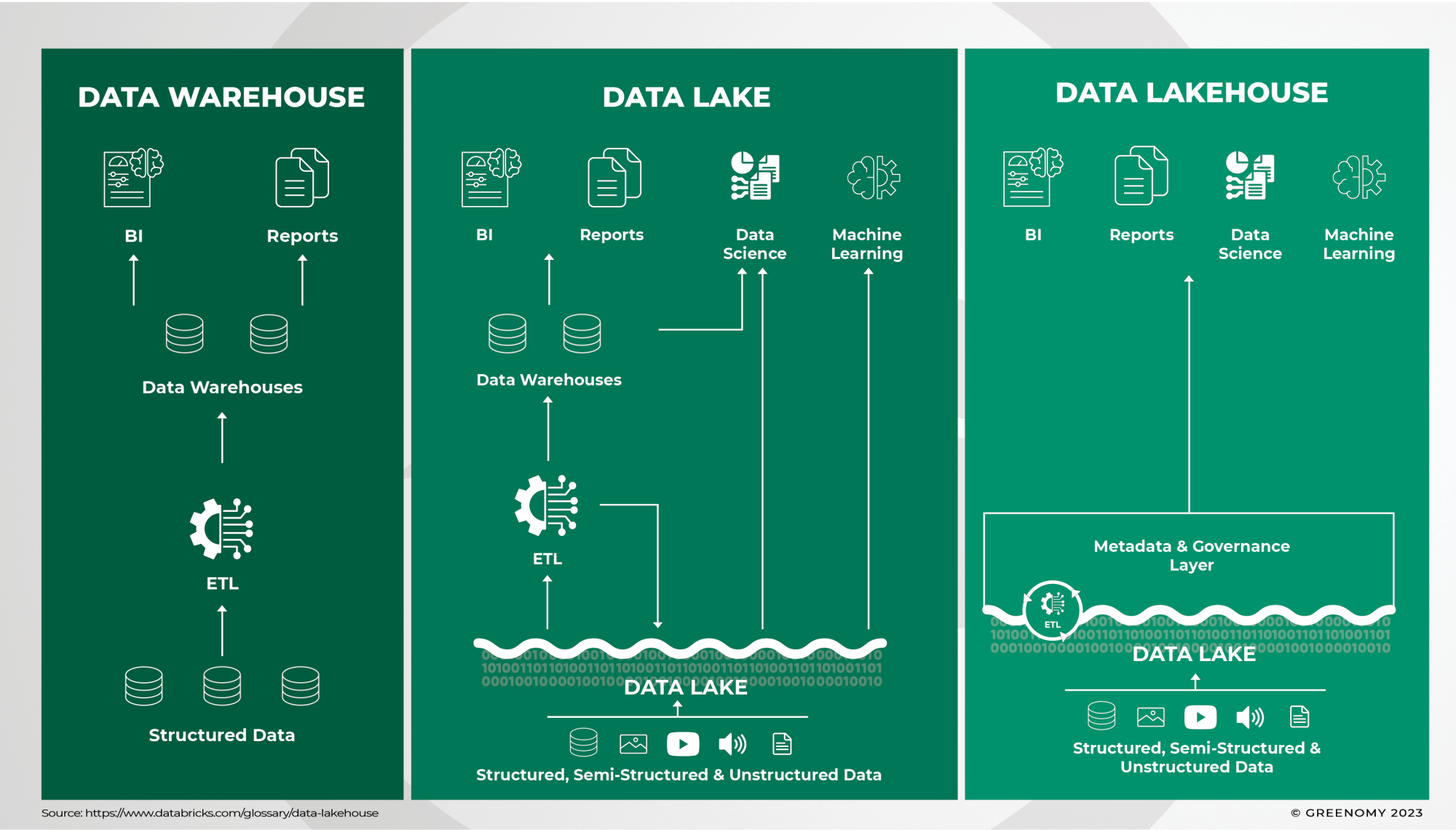
Data Lakes and ESG Reporting
Ensuring Data Quality, Integrity, and Consistency
The EU Sustainable Finance reporting requirements for corporates — the CSRD and EU Taxonomy — are a particularly challenging set of regulations. With over 3,700 ESG data points that need to be reported, and derived from a wide variety of sources, gathering and organising such a significant volume of information requires substantial effort and resources. Data lakes offer a solution to these challenges by ensuring data quality, verifying data integrity, and addressing potential gaps or inconsistencies.
Data lakes can accommodate diverse types of ESG data, ranging from structured employee data from a dedicated software, to unstructured energy consumption data in various formats, such as PDF documents. Raw ESG data can also be pulled from Internet Of Things (IoT) sensors, which monitor energy consumption or waste management — some of the KPIs to be included in the CSRD reporting. This unassorted input data — anonymised if needed — is cleansed and standardised into the data lake, through manual, semi-automated, or automated processes.
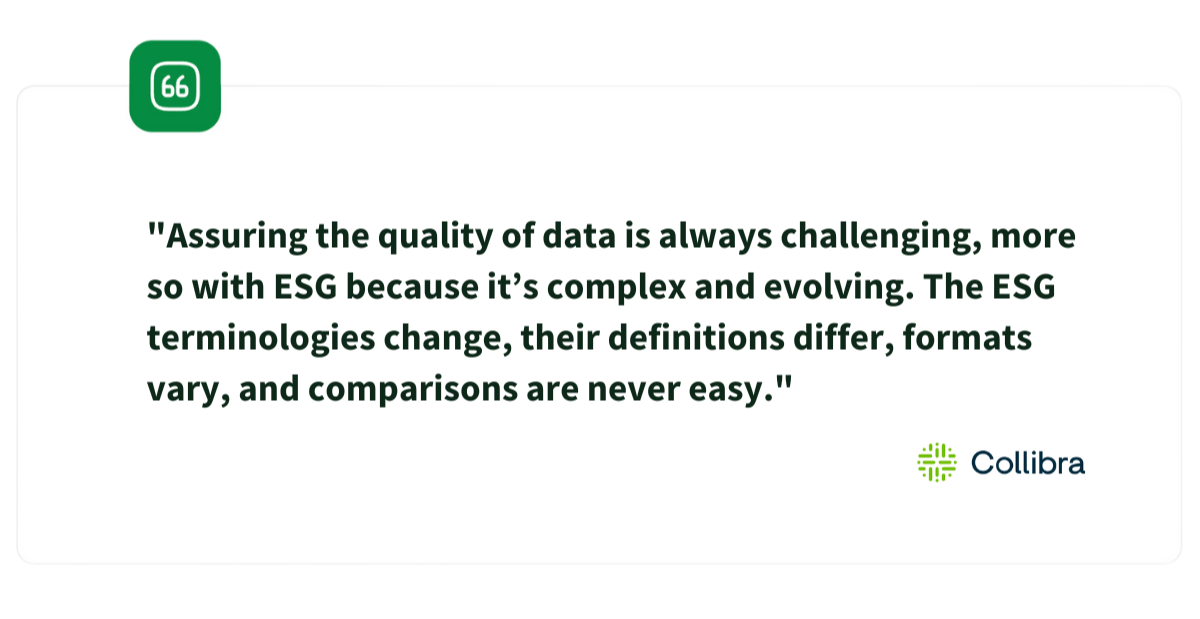
Issuing Audit-Ready Reports
The data is then mapped against the ESG data model of the reporting platform, built to provide the relevant ESG Key Performance Indicators (KPIs) matching the regulatory data points. Regulation updates or future reporting requirements can easily be accommodated, and simply need to be cross-tagged onto the existing input data model — therefore avoiding the duplication of data collection and ingestion work.
The Data Lake architecture ensures complete traceability of ESG KPIs, allowing companies to track data back to their source systems, and enhancing transparency and auditability. This audit trail feature is key to complying with the limited assurance requirement from the CSRD.
To learn more about the CSRD, see our dedicated article on the Essentials of the CSRD.
Applying Data Lakes to CSRD: An HR Data Use Case
While the CSRD requires close to 50,000 companies to issue a sustainability report subject to limited assurance, the ESG KPIs to be reported and their format are detailed in the European Sustainability Reporting Standards (ESRS). The latest draft of the ESRS was released in early June, and the rest of the standards are expected in 2024. In this section, we will explain how a data lake answers the challenges of regulatory frameworks that keep being updated or supplemented over time.
To illustrate this, let’s look at some of the Human Resources (HR) KPIs to be reported under ESRS S1 (“Social” pillar). HR data is typically sourced from various structured (i.e.: ERPs, specialised HR systems), semi-structured (i.e.: employee lists in Excel format), and unstructured (i.e.: CVs, payslips in PDF format) data sources within an organisation. Additionally, different entities within the same corporate group may utilise distinct classification systems, resulting in data disparity.

If we look closer into the example of the gender pay gap, disclosed under ESRS S1, lists of employees per gender, function, and salary level may come from different sources and under different formats. In addition to the use of various HR systems, companies also have to report following different methodologies based on national requirements. The ESG Data Library transforms them into a standardised global list of employees per gender, function, and salary range. This data flows into a data model built against the ESRS reporting templates — using here the appropriate gender pay gap calculation methodology outlined in ESRS S1.
The work put in providing a unified, validated input data model can be leveraged in the future for further reporting obligations — i.e.: the second set of sector-specific ESRS, new EU regulations, and other international frameworks which the company may choose/need to report on. If, say, a requirement of disclosing the % of LGBTQ+ people in management is added, the existing data model can easily be extended: the relevant business data owners simply add a new field in the ESG Data Library with automatic inclusion in the framework disclosure.
One Step Further: Implementing ESG Data Solution for Business Intelligence (BI) and Artificial Intelligence (AI)
We have seen that ESG data models provide a data management architecture supporting companies in the long run, whether they go through organisational changes or face new disclosure requirements. Beyond ESG compliance, data solutions offer broader tools to help transform a company. Having structured ESG data allows companies to seamlessly connect their Business Intelligence (BI) tools to gain actionable insights that improve business decisions. As well using integrated artificial intelligence (AI) tools, they can offer predictive ESG analytics and advanced use cases. This can be further enabled using machine learning (ML) evidence extraction from PDF documents.
As organisations work on their transition strategy, they can use the data solution to model both short- and long-term sustainability goals, align initiatives across their operations, and make informed decisions based on an integrated understanding of ESG, finance, and operations.
While the CSRD requires companies to reassess their approach to ESG reporting, the positive outcomes of streamlined CSRD reporting are multifold: improved stakeholder engagement, investor confidence, and competitive advantage.
Greenomy’s ESG Data Library: A Comprehensive Data Collection Model for CSRD Reporting
Implementing an ESG Data Library offers a comprehensive solution to the challenges of collecting and standardising data for sustainability reporting. This architecture ensures data flexibility, integrity, and traceability while enabling future adaptability. Leveraging the data lakes’ capabilities, organisations can not only meet the regulatory requirements but also drive transformative decision-making, and advance their sustainability goals.
Conscious of the need for companies to have a centralised platform for both data management and regulatory reporting, Greenomy developed its ESG Data Library, embedded in the front end of the Company Portal. The platform allows you to feed the data model with granular data from your system - via customisable predefined import templates or our 100+ data connectors from your IT systems. With the CSRD’s ESRS mapped into a user-friendly interface, we then guide you through your reporting exercise to issue your CSRD report ready for disclosure and audit. Greenomy works with its data consultant partners to integrate its data model into your existing IT landscape.
Additionally, our solution was recognised with as runners-up in the SWIFT Innotribe Hackathon 2023, SWIFT first prize for Sustainability at SIBOS in 2022 in Amsterdam as well as winning first prize in Milan in 2021 at the G20 TechSprint competition for Sustainable Finance solutions.
Contact our ESG Data Experts to discover how Greenomy can help you streamline our CSRD/EU Taxonomy reporting.